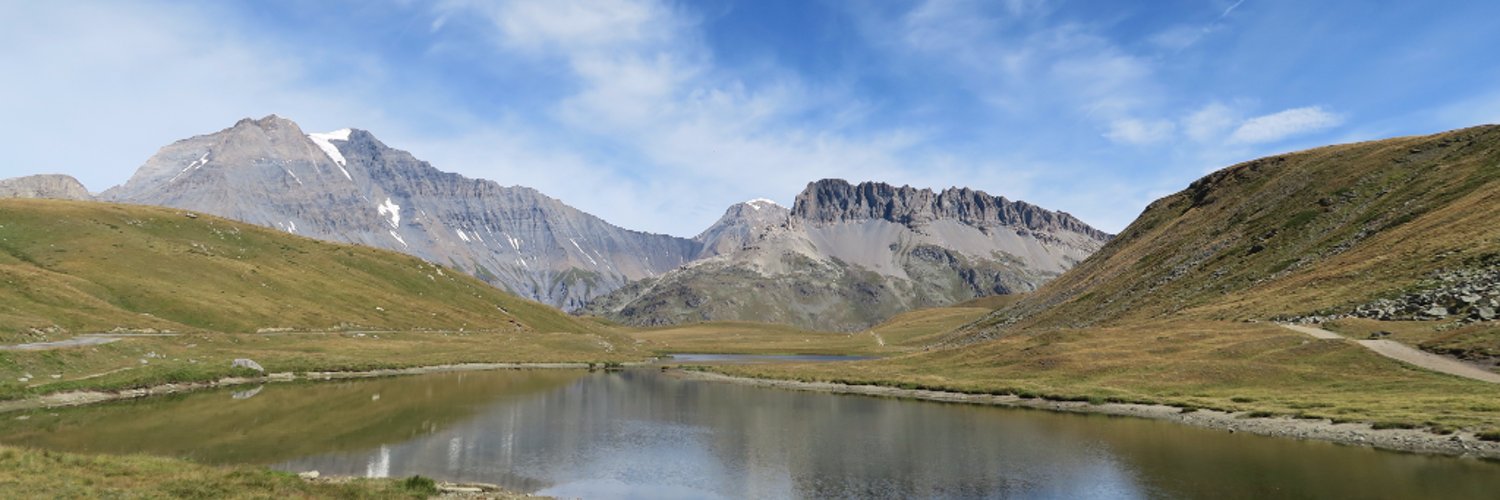
Gutu and Paraschiv PhD Thesis Committees
On Thursday 28th, Nov., I’ll be attending the PhD thesis committee of two students from University ‘Politehnica’ of Bucharest, Gabriel Gutu and Ionut Paraschiv, supervised by Stefan Trausan-Matu.
Gabriel Gutu‘s thesis is entitled “Discourse Analysis based on Semantic Modelling and Textual Complexity” and aims at extending some ReaderBench‘s functionalities in the domain of CSCL discussion analysis.
Ionut Paraschiv’s thesis (Semantic Meta-Annotation and Comprehension Modeling) also adds features to ReaderBench, in comprehension modeling and scientometrics.
For more information, read below their summaries.
Gabriel Gutu thesis’ summary
The exponential growth of digital documents, together with the necessity for analysis and extraction of valuable information within them, bring routine work for people. The opportunity for development of automated discourse analysis services and techniques leads to automation of laborious operations. In the long run, the transferring of tiresome operations into computerized systems would allow people to focus on “high level” assignments that lead to interesting ideas and provide the means to extract thoughts and understandings that are currently hardly interpreted by computers.
Discourse analysis refers to the extraction of relevant information from documents by using techniques of analysis known in scientific literature as Natural Language Processing. The services presented in this thesis make use of recent advancements in the field by integrating semantic models and textual complexity factors. Semantic models allow the mapping of documents into mathematical representations that provide comparison and scoring for units of texts, be them either simple words, sentences, paragraphs or even entire documents. Of the semantic models, the thesis relies on Latent Semantic Analysis, Latent Dirichlet Allocation and the more recent Word2vec. The WordNet ontology is the lexicon used as alternative to semantic models. Compared to semantic models, a lexicon expresses “more natural” relations between units of texts because of relying on a dictionary and using relations between words that are created in collaboration with linguists.
The experiments were performed by extending ReaderBench, a multi-lingual Natural Language Processing open-source framework. Two directions were followed: 1) analysis of Computer Supported Collaborative Learning (CSCL) chat conversations; 2) automation of processes of discourse analysis through mechanisms adaptable to various scenarios relying on textual content. The studies performed on CSCL conversations targeted the idea of developing an automated mechanism of detection of implicit links, facility that is missing in chat platforms. By integrating such a mechanism, the outlined relations between utterances may ease processes like detection of topics, voices or lexical chains. The researches performed on documents included automated classification of documents, assessment of documents’ quality or automated scoring of students’ assignments in a Massive Open Online Course platform. The mechanisms were validated on real world data extracted. Services were exposed through an Application Programming Interface.
The author of this thesis beliefs that the presented experiments could provide ideas for future studies and could ease the involved work by allowing researchers to focus on their topics while relying on the validated mechanisms by using the implemented services made available through the open-source ReaderBench framework.
Ionut Paraschiv thesis’ summary
Each domain, alongside its knowledge base, changes over time and each period is centered on specific topics that emerge from different ongoing research projects. A researcher’s daily activities usually involve the study of new papers and using the information for building solutions and observing how the domain evolves. Since the retrieval of documents from the Internet can lead to large data flows, it is important to consider other approaches for a more comprehensive analysis of the domain. In this context, the Semantic Meta Annotations focus on building a scalable paper annotation system that automatically retrieves papers on a given topic and tags them, to make the exploration phase of the research literature substantially easier.
Evolution is based on leveraging existing knowledge, researches and tools to test other ideas. A researcher needs to read many textual materials, which are many times cluttered with irrelevant information. Thus, the focus of our research is shifted towards understanding the way in which humans comprehend texts. Reading is a complex cognitive process which has been the subject of many studies throughout the years. It is one of the oldest ways for learners to acquire new information and to consolidate existing knowledge, representing a key evolutionary element. Each textual material contains facts and topics that activate existing concepts from the reader’s prior knowledge (memory). The Comprehension Model describes an automated method that analyzes the way in which readers potentially assimilate and conceptualize new text information, which is a novel alternative for indexing and meta-annotating textual corpora. Creating such a method is a challenge, as it requires using a computational knowledge base, parsing unstructured textual materials and linking concepts using various heuristics and semantic similarity measures.
Our research focuses on the semantic analysis of unstructured textual materials by using Natural Language Processing techniques and models such as Latent Semantic Analysis, Latent Dirichlet Allocation, Word2Vec or semantic distances within lexicalized ontologies, i.e., WordNet. Within the experiments focused on semantic meta annotations, these distances are combined with other metrics such as co-citation analysis or co-authorship, thus creating the basis of several interactive and exploratory visual graphs that offer a better domain overview within a scalable infrastructure. In the second experiment, our focus is shifted towards describing an automatic comprehension modelling technique that analyzes using computational representations and algorithms the reading process. Our goal was to create a set of methods and tools to help researchers in their daily work to easily retrieve and understand textual materials.